Publications by Tags
Selected Papers Compression, Equivariance, Extrapolation, Generalization, Information Theory, Invariance, Meta Learning, NLP, Representation Learning, Robustness, Self-Supervised Learning, Time Series, Uncertainty, VisionSelected Papers
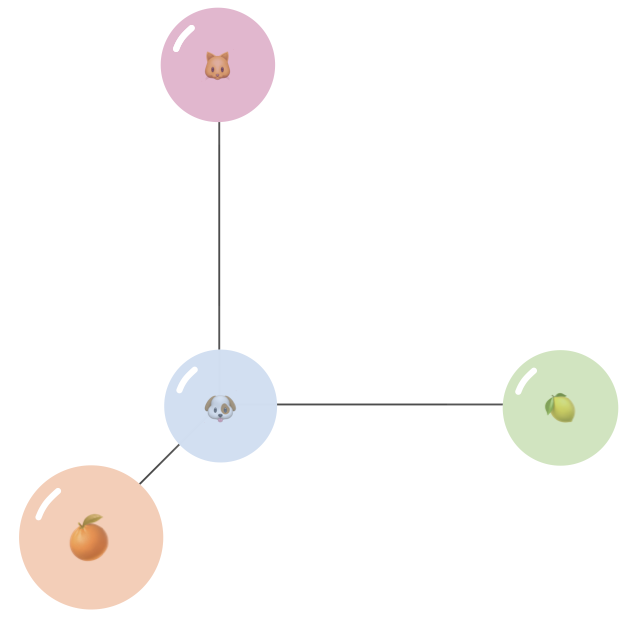
Y. Dubois, T. Hashimoto, S. Ermon, P. Liang
NeurIPS 2022TLDR: We characterize idealized self-supervised representations, which leads to actionable insights for improving SSL algorithms.
Selected Papers Invariance, Representation Learning, Self-Supervised Learning, Vision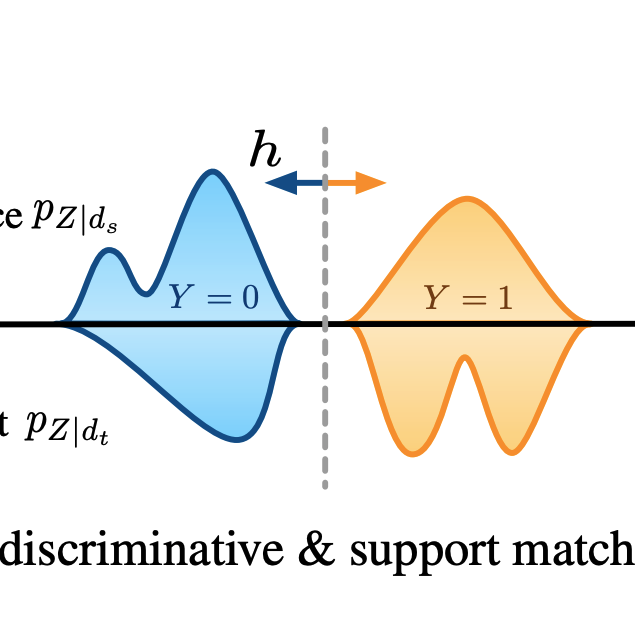
Y Ruan*, Y. Dubois*, C. J. Maddison
ICLR 2021TLDR: We give a simple variational objective whose optima are exactly the set of representations that are robust under covariate shift.
Selected Papers Information Theory, Invariance, Representation Learning, Robustness, Self-Supervised Learning, Vision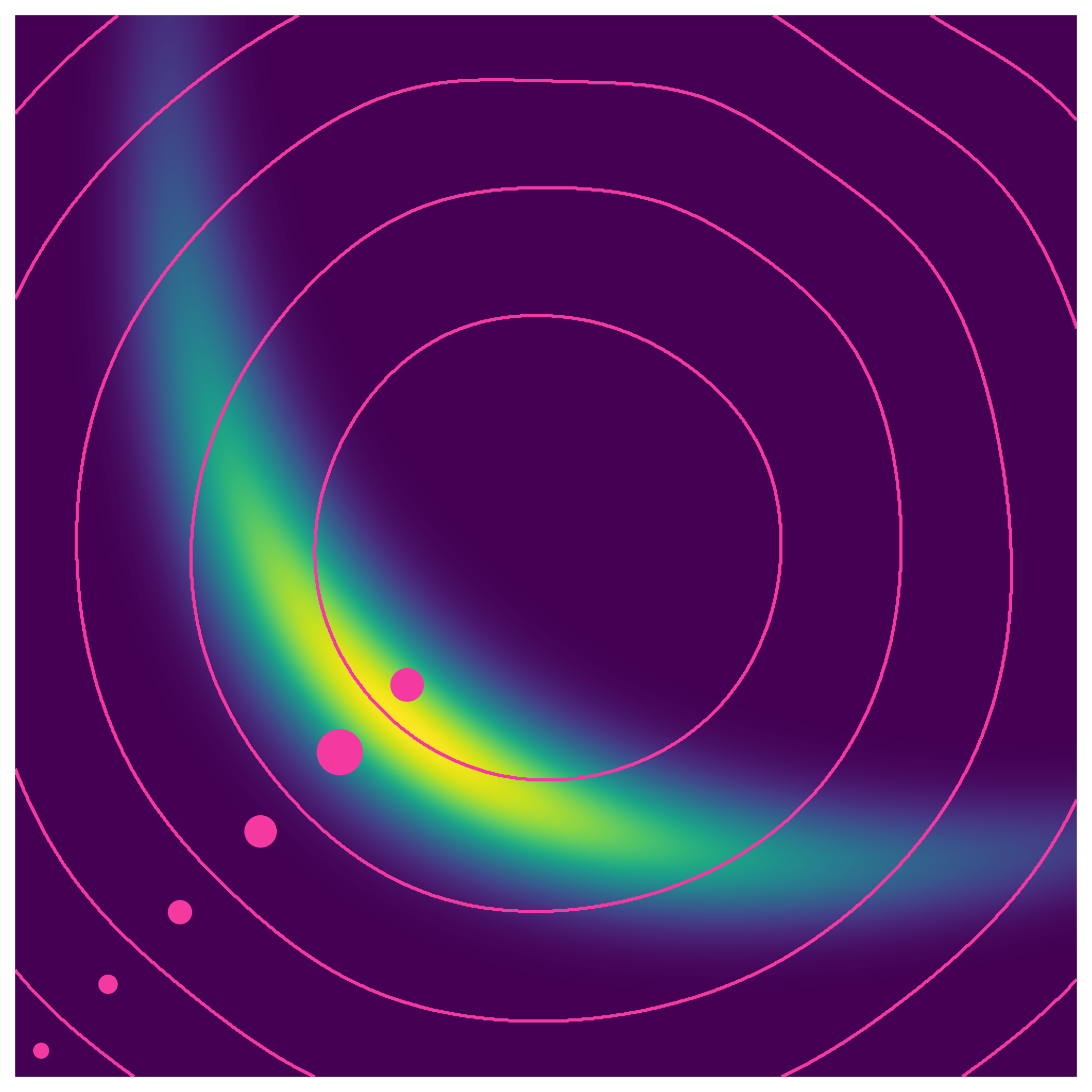
Y. Dubois, B. Bloem-Reddy, K. Ullrich, C. J. Maddison
NeurIPS 2021 Spotlight Presentation 🎉TLDR: We formalize compression with respect to ML algorithms rather than human perception.
Selected Papers Compression, Information Theory, Invariance, Representation Learning, Self-Supervised Learning, Vision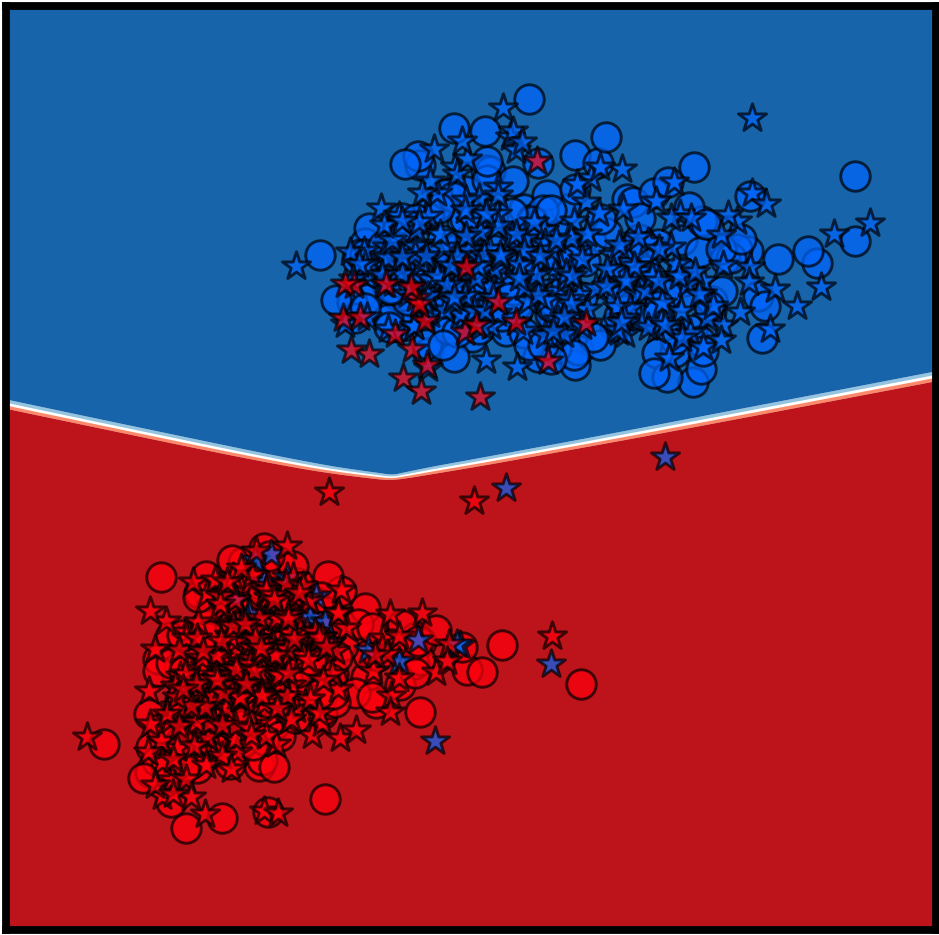
Y. Dubois, D. Kiela, D. J. Schwab, R. Vedantam
NeurIPS 2020 Spotlight Presentation 🎉TLDR: We characterize and approximate optimal representations for supervised learning.
Selected Papers Generalization, Information Theory, Representation Learning, VisionCompression
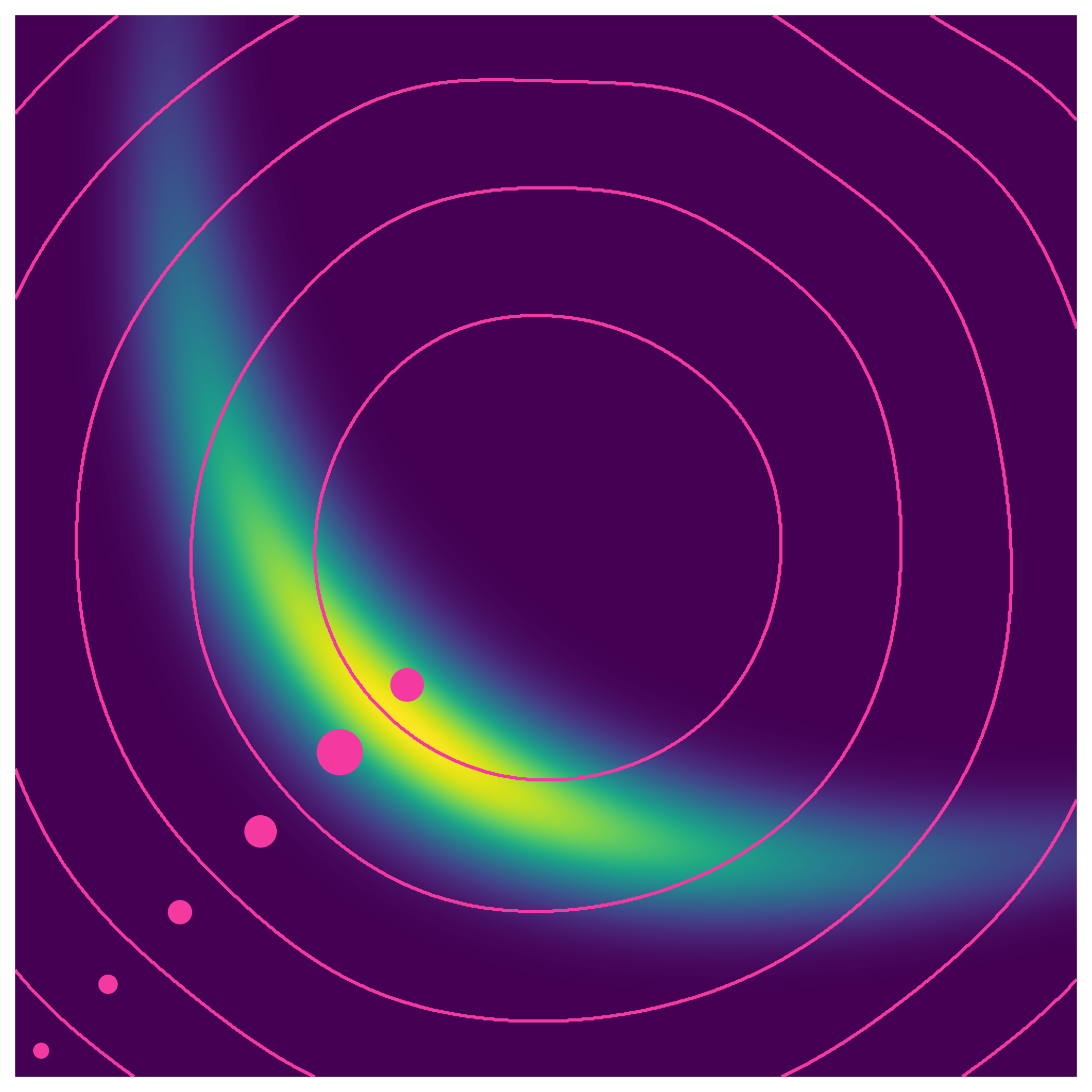
Y. Dubois, B. Bloem-Reddy, K. Ullrich, C. J. Maddison
NeurIPS 2021 Spotlight Presentation 🎉TLDR: We formalize compression with respect to ML algorithms rather than human perception.
Selected Papers Compression, Information Theory, Invariance, Representation Learning, Self-Supervised Learning, VisionEquivariance
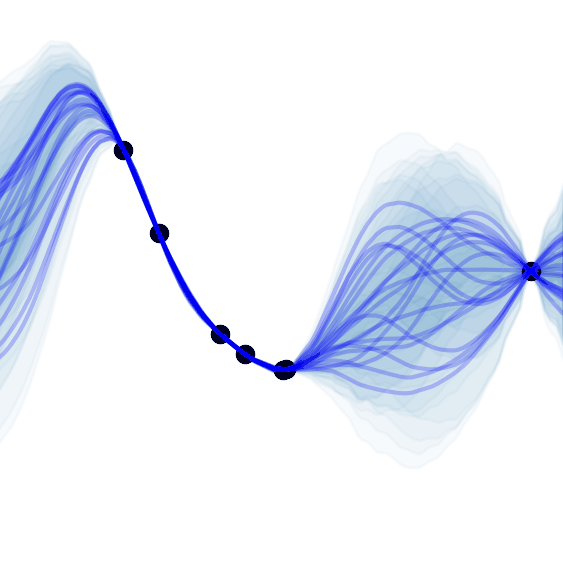
A. Y. K. Foong*, W. P. Bruinsma*, J. Gordon*, Y. Dubois, J. Requeima, R. E. Turner
NeurIPS 2020TLDR: We propose a translation equivariant (latent) neural process.
Equivariance, Extrapolation, Meta Learning, Time Series, Uncertainty, Vision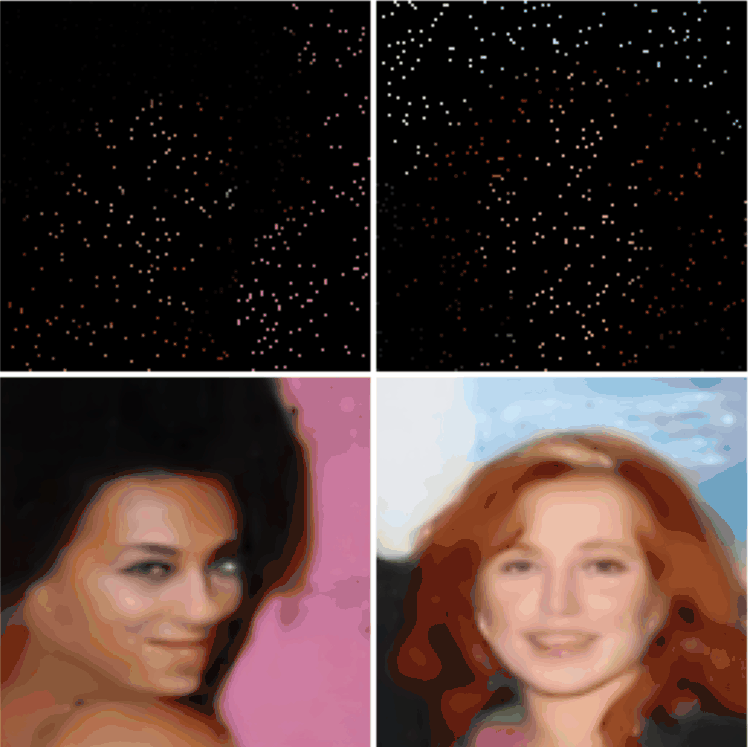
J. Gordon*, W. P. Bruinsma*, A. Y. K. Foong, J. Requeima,Y. Dubois, R. E. Turner
ICLR 2020 Oral Presentation 🎉TLDR: We propose a translation equivariant conditional neural process.
Equivariance, Extrapolation, Meta Learning, Time Series, Uncertainty, VisionExtrapolation
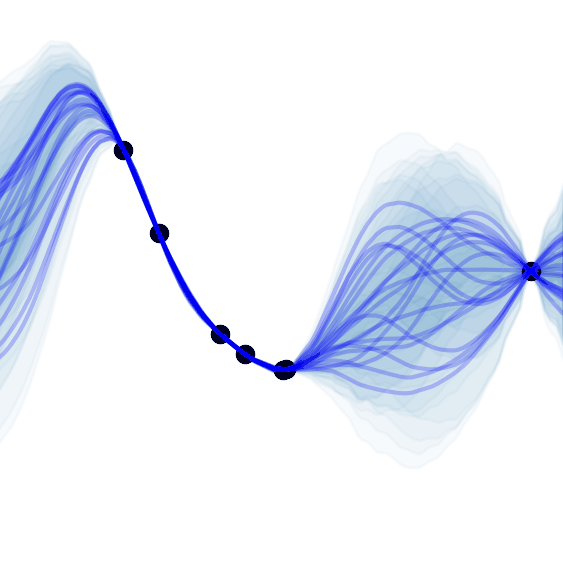
A. Y. K. Foong*, W. P. Bruinsma*, J. Gordon*, Y. Dubois, J. Requeima, R. E. Turner
NeurIPS 2020TLDR: We propose a translation equivariant (latent) neural process.
Equivariance, Extrapolation, Meta Learning, Time Series, Uncertainty, Vision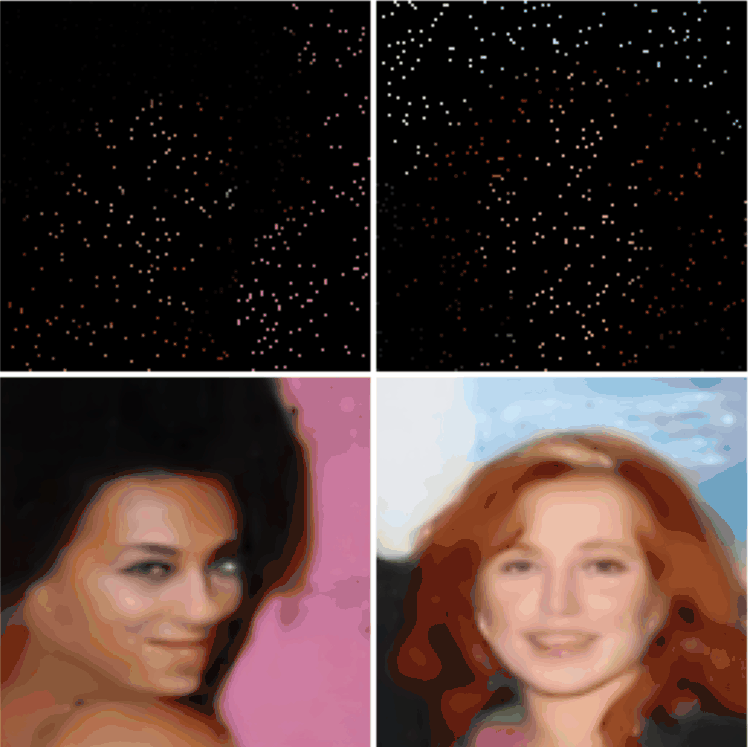
J. Gordon*, W. P. Bruinsma*, A. Y. K. Foong, J. Requeima,Y. Dubois, R. E. Turner
ICLR 2020 Oral Presentation 🎉TLDR: We propose a translation equivariant conditional neural process.
Equivariance, Extrapolation, Meta Learning, Time Series, Uncertainty, Vision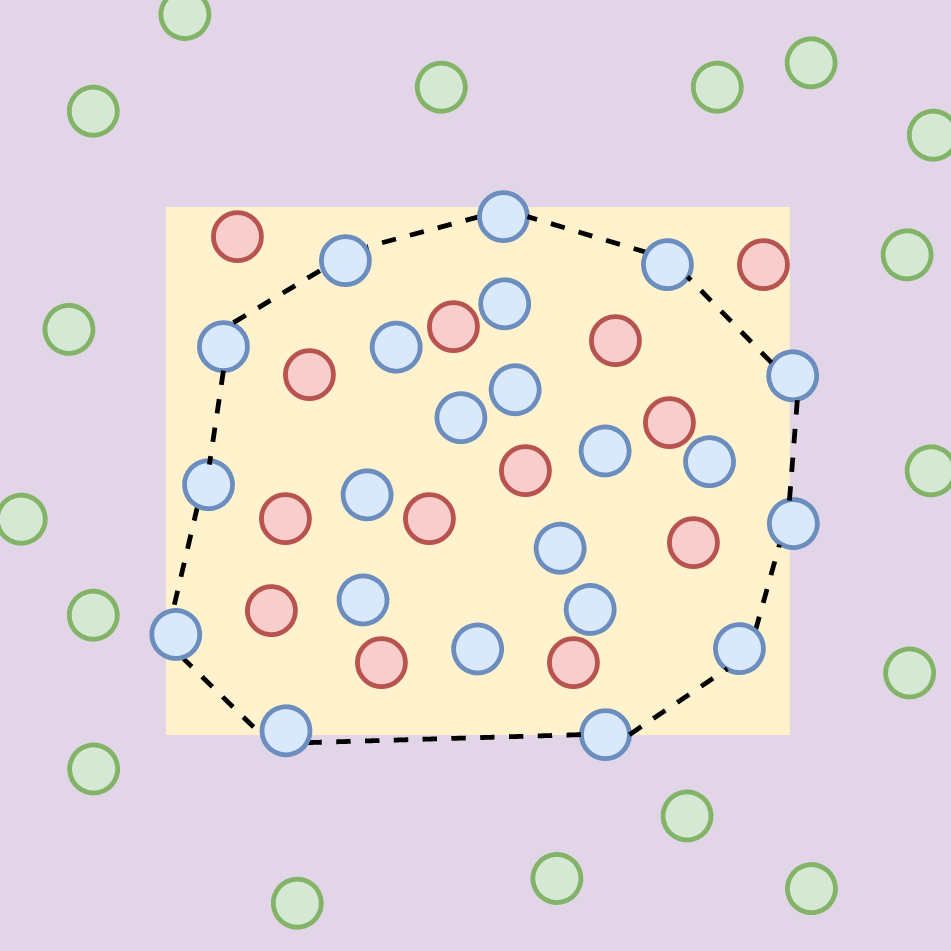
Y. Dubois, Gautier Dagan, Dieuwke Hupkes, Elia Bruni
ACL 2020TLDR: We propose an attention that improves extrapolation capacity of neural NLP models.
Extrapolation, NLP, RobustnessGeneralization
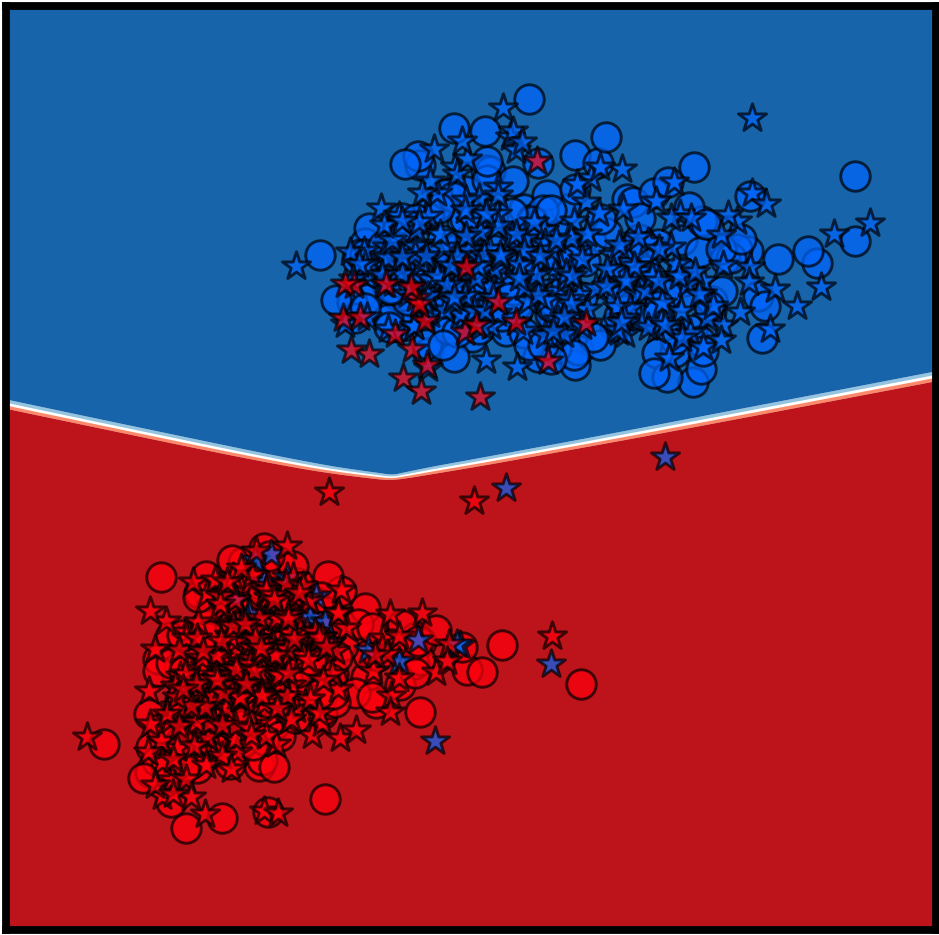
Y. Dubois, D. Kiela, D. J. Schwab, R. Vedantam
NeurIPS 2020 Spotlight Presentation 🎉TLDR: We characterize and approximate optimal representations for supervised learning.
Selected Papers Generalization, Information Theory, Representation Learning, VisionInformation Theory
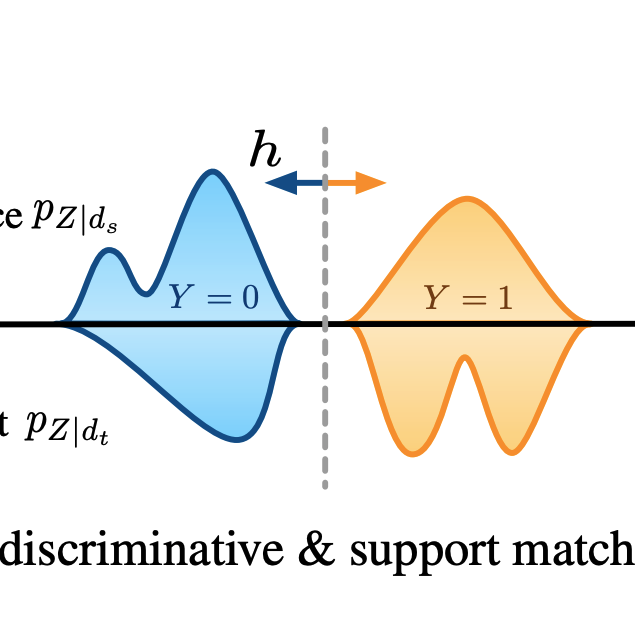
Y Ruan*, Y. Dubois*, C. J. Maddison
ICLR 2021TLDR: We give a simple variational objective whose optima are exactly the set of representations that are robust under covariate shift.
Selected Papers Information Theory, Invariance, Representation Learning, Robustness, Self-Supervised Learning, Vision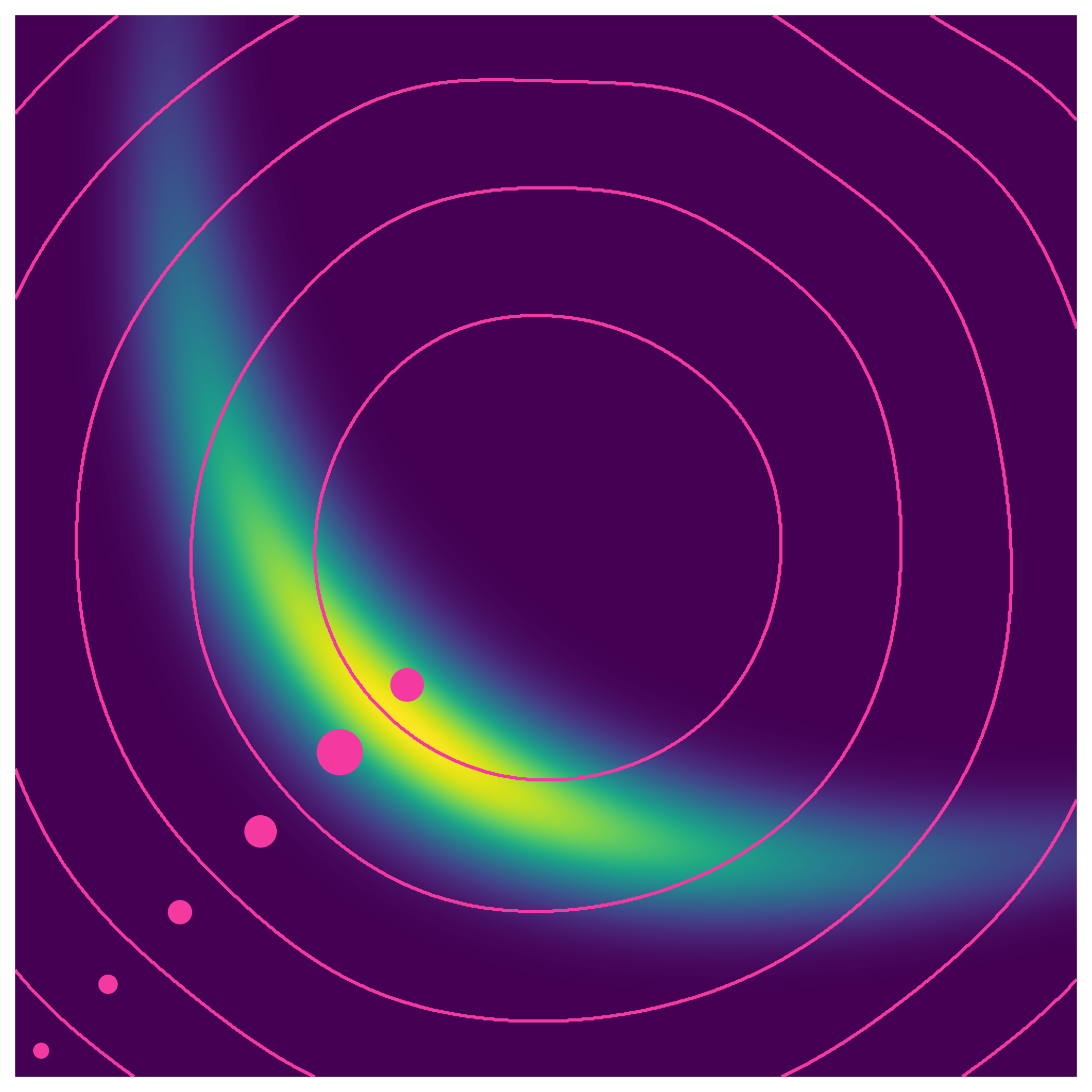
Y. Dubois, B. Bloem-Reddy, K. Ullrich, C. J. Maddison
NeurIPS 2021 Spotlight Presentation 🎉TLDR: We formalize compression with respect to ML algorithms rather than human perception.
Selected Papers Compression, Information Theory, Invariance, Representation Learning, Self-Supervised Learning, Vision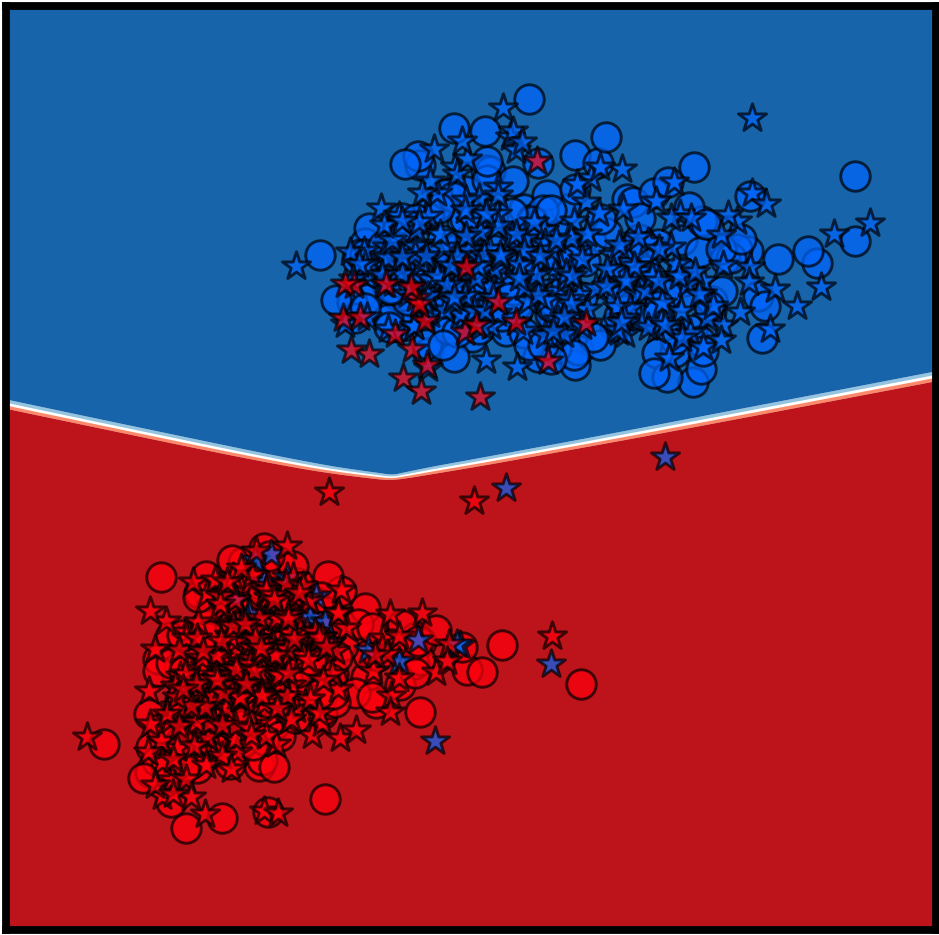
Y. Dubois, D. Kiela, D. J. Schwab, R. Vedantam
NeurIPS 2020 Spotlight Presentation 🎉TLDR: We characterize and approximate optimal representations for supervised learning.
Selected Papers Generalization, Information Theory, Representation Learning, VisionInvariance
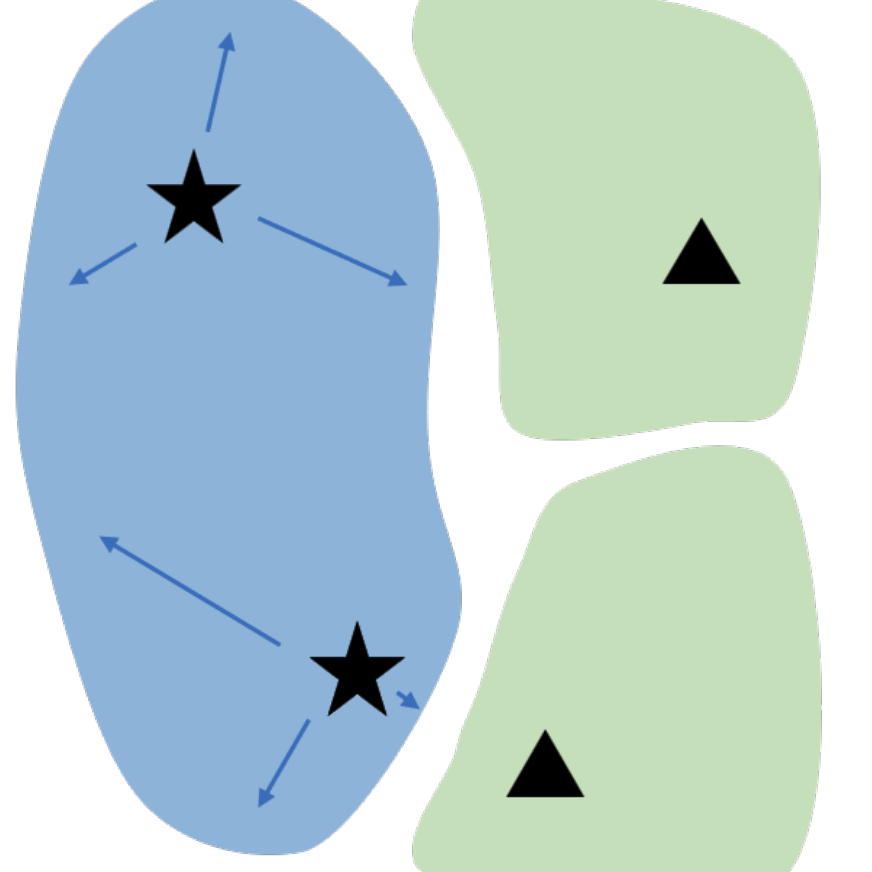
N. Miao, E. Mathieu, Y. Dubois, T. Rainforth, Y. W. Teh, A. Foster, H. Kim
ArxivTLDR: We introduce a method for automatically learning input-specific augmentations from data.
Invariance, Vision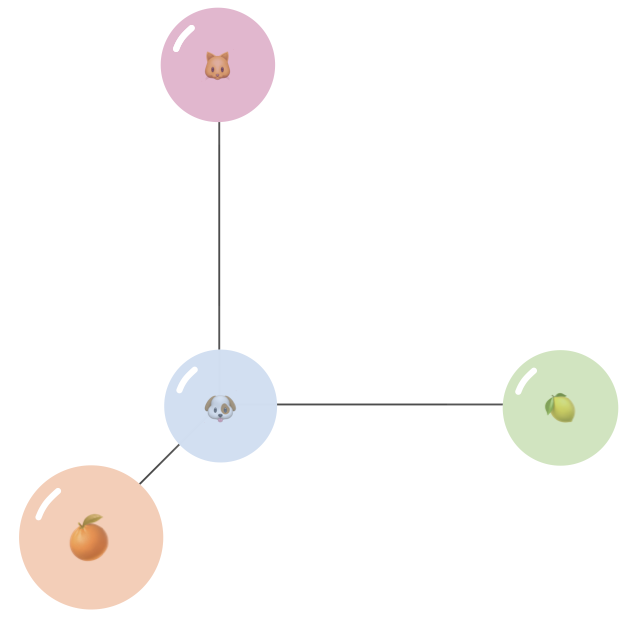
Y. Dubois, T. Hashimoto, S. Ermon, P. Liang
NeurIPS 2022TLDR: We characterize idealized self-supervised representations, which leads to actionable insights for improving SSL algorithms.
Selected Papers Invariance, Representation Learning, Self-Supervised Learning, Vision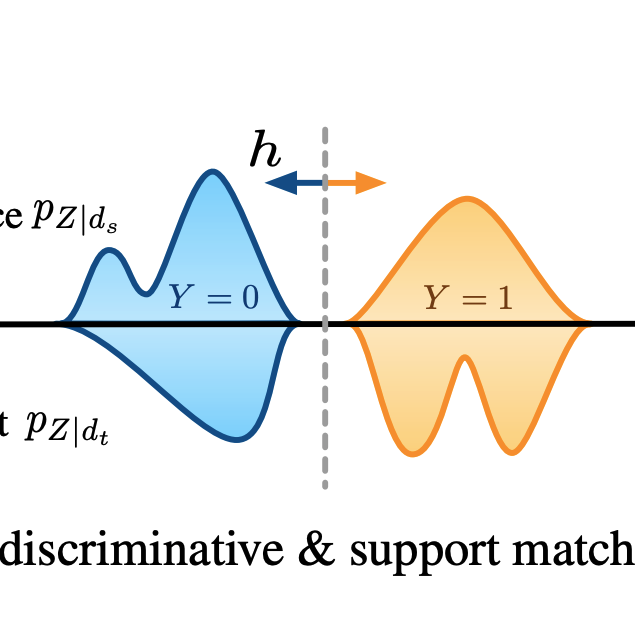
Y Ruan*, Y. Dubois*, C. J. Maddison
ICLR 2021TLDR: We give a simple variational objective whose optima are exactly the set of representations that are robust under covariate shift.
Selected Papers Information Theory, Invariance, Representation Learning, Robustness, Self-Supervised Learning, Vision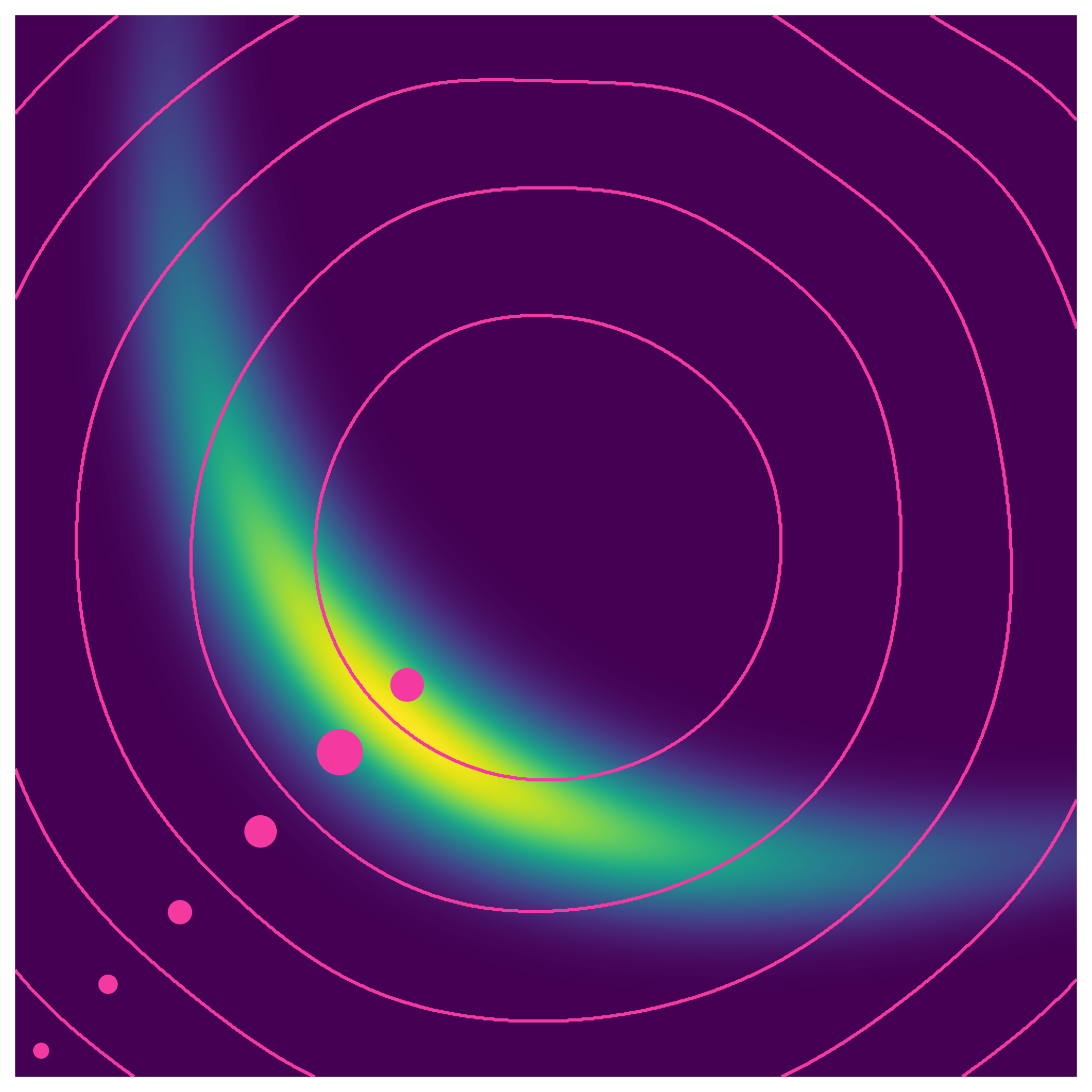
Y. Dubois, B. Bloem-Reddy, K. Ullrich, C. J. Maddison
NeurIPS 2021 Spotlight Presentation 🎉TLDR: We formalize compression with respect to ML algorithms rather than human perception.
Selected Papers Compression, Information Theory, Invariance, Representation Learning, Self-Supervised Learning, VisionMeta Learning
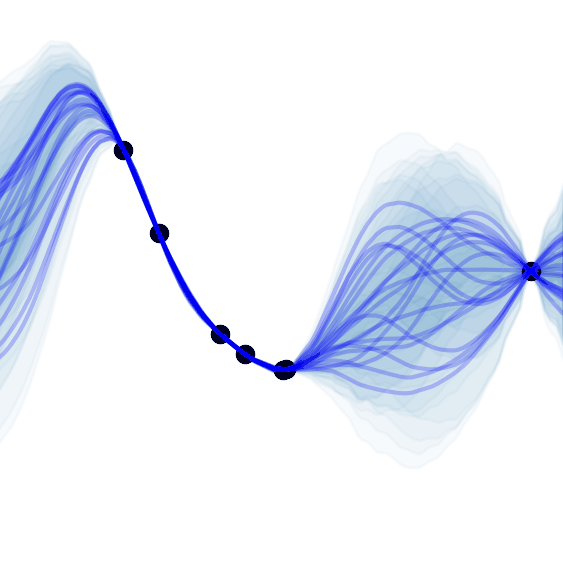
A. Y. K. Foong*, W. P. Bruinsma*, J. Gordon*, Y. Dubois, J. Requeima, R. E. Turner
NeurIPS 2020TLDR: We propose a translation equivariant (latent) neural process.
Equivariance, Extrapolation, Meta Learning, Time Series, Uncertainty, Vision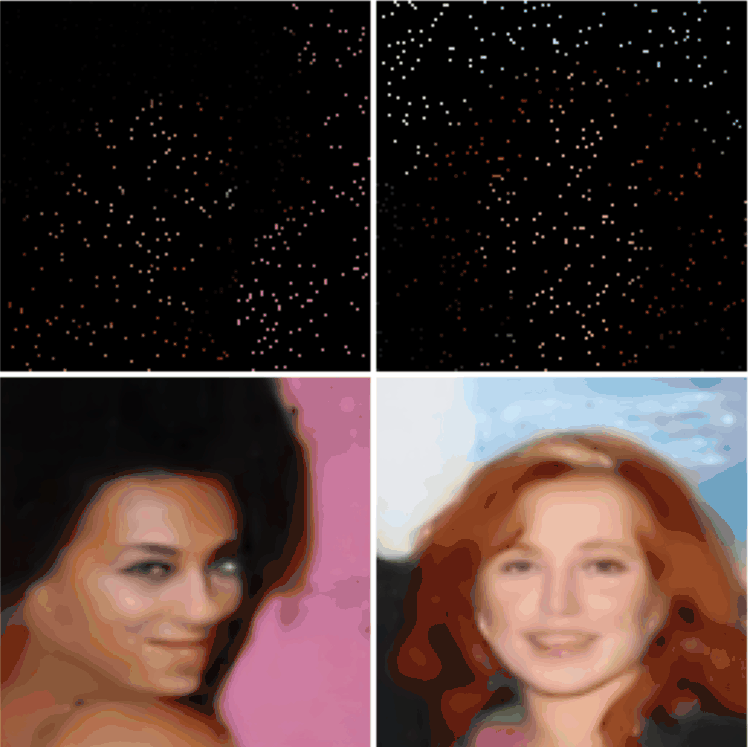
J. Gordon*, W. P. Bruinsma*, A. Y. K. Foong, J. Requeima,Y. Dubois, R. E. Turner
ICLR 2020 Oral Presentation 🎉TLDR: We propose a translation equivariant conditional neural process.
Equivariance, Extrapolation, Meta Learning, Time Series, Uncertainty, VisionNLP
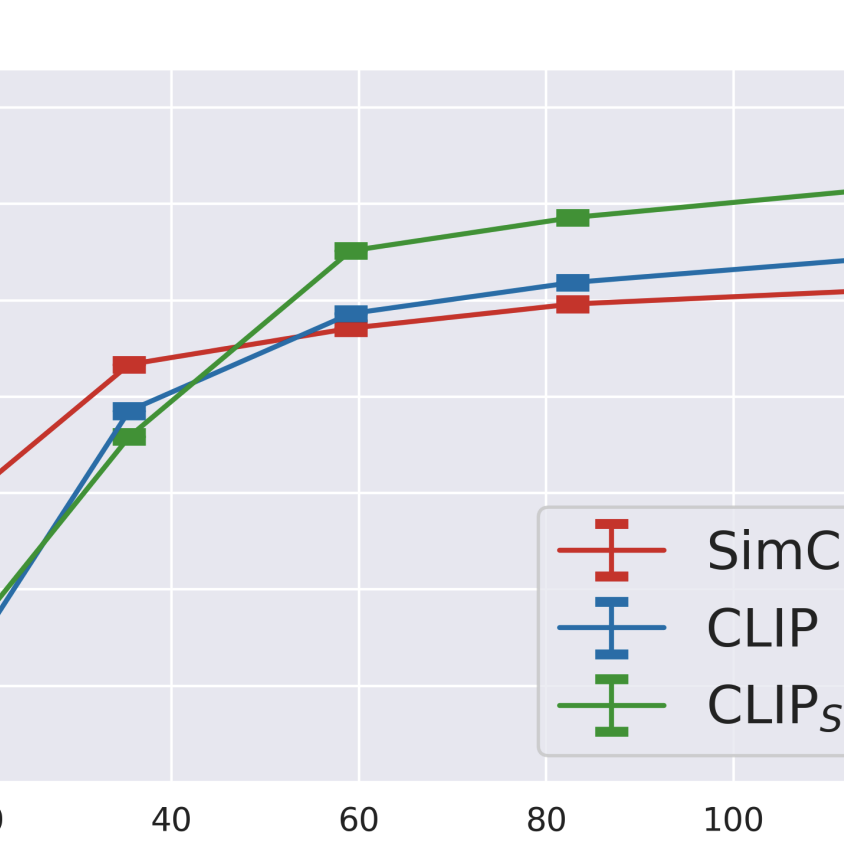
S. Santurkar, Y. Dubois, R. Taori, P. Liang, T. Hashimoto
ArxivTLDR: Our work performs a systematic investigation into whether additional language supervision (in CLIP) helps models learn more transferrable representations.
NLP, Representation Learning, Self-Supervised Learning, Vision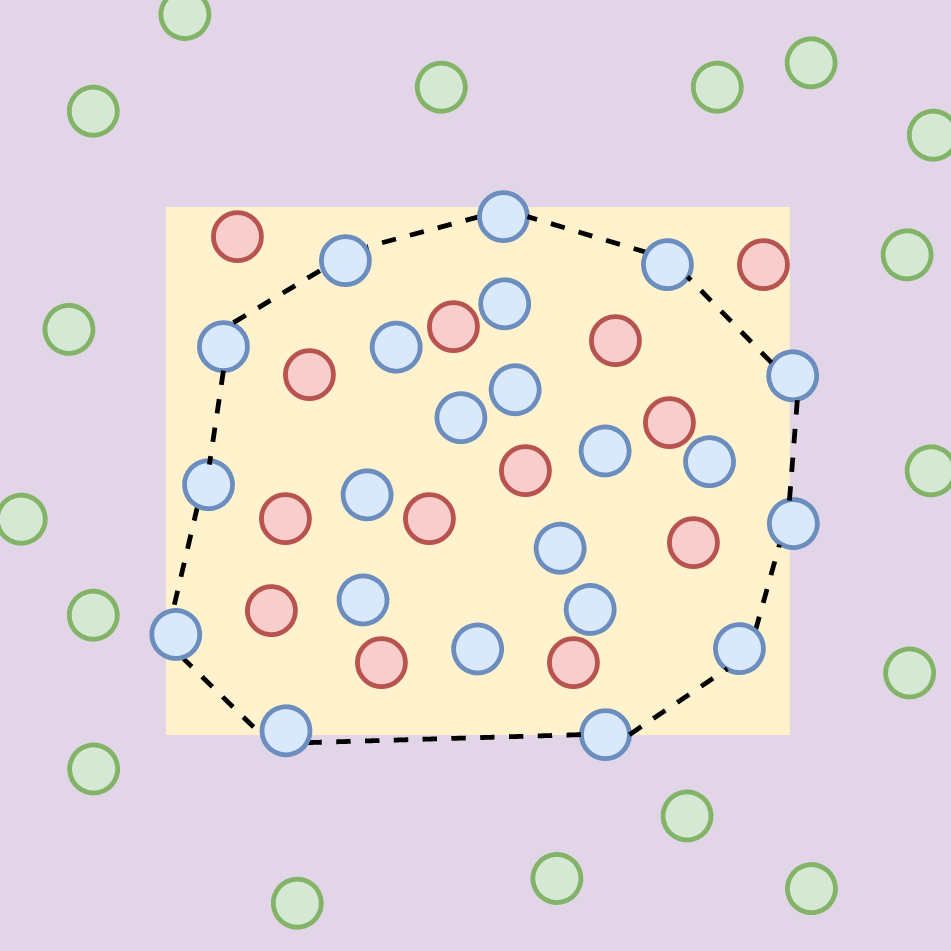
Y. Dubois, Gautier Dagan, Dieuwke Hupkes, Elia Bruni
ACL 2020TLDR: We propose an attention that improves extrapolation capacity of neural NLP models.
Extrapolation, NLP, RobustnessRepresentation Learning
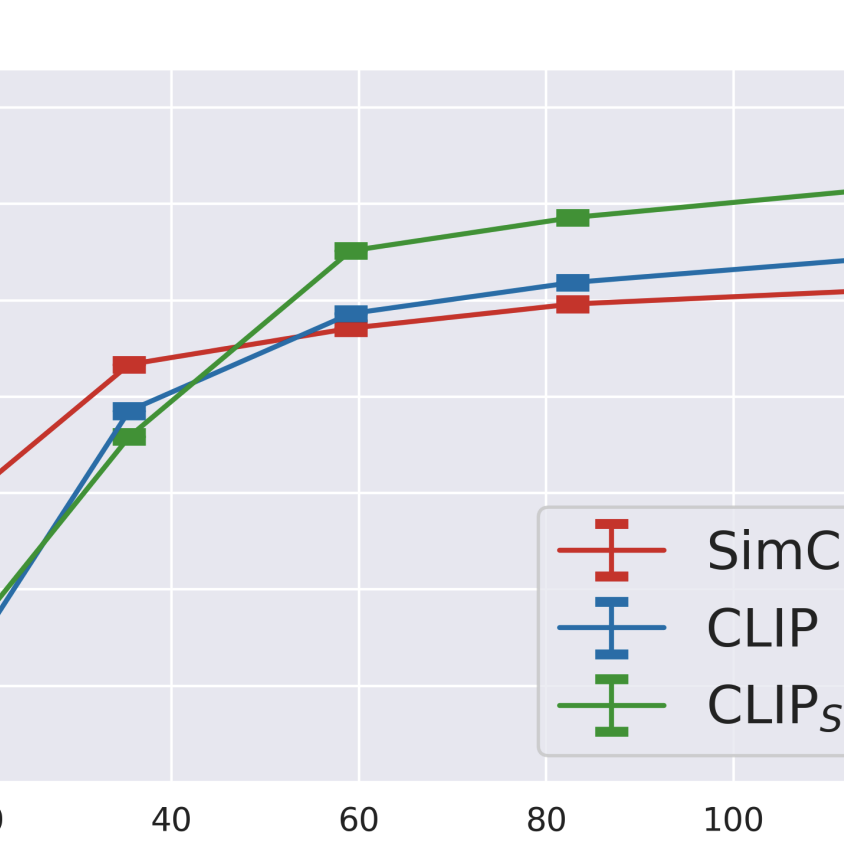
S. Santurkar, Y. Dubois, R. Taori, P. Liang, T. Hashimoto
ArxivTLDR: Our work performs a systematic investigation into whether additional language supervision (in CLIP) helps models learn more transferrable representations.
NLP, Representation Learning, Self-Supervised Learning, Vision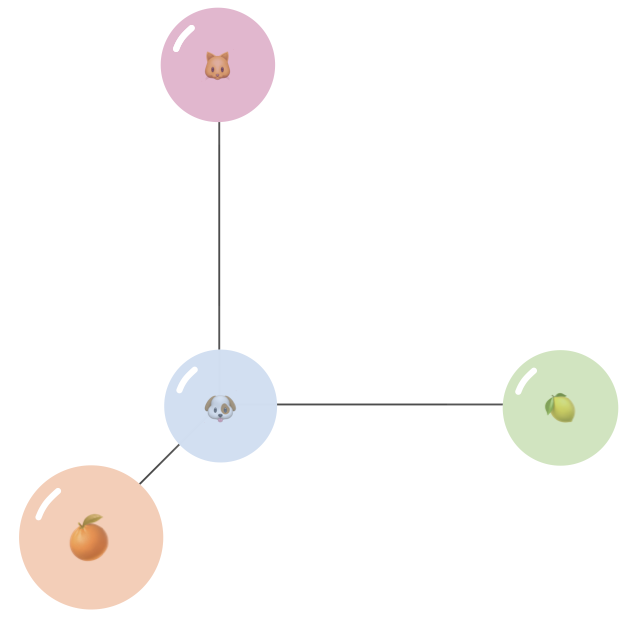
Y. Dubois, T. Hashimoto, S. Ermon, P. Liang
NeurIPS 2022TLDR: We characterize idealized self-supervised representations, which leads to actionable insights for improving SSL algorithms.
Selected Papers Invariance, Representation Learning, Self-Supervised Learning, Vision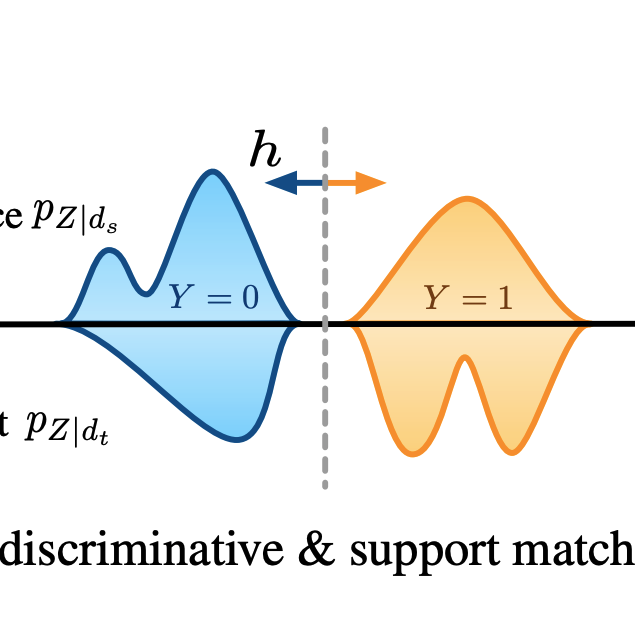
Y Ruan*, Y. Dubois*, C. J. Maddison
ICLR 2021TLDR: We give a simple variational objective whose optima are exactly the set of representations that are robust under covariate shift.
Selected Papers Information Theory, Invariance, Representation Learning, Robustness, Self-Supervised Learning, Vision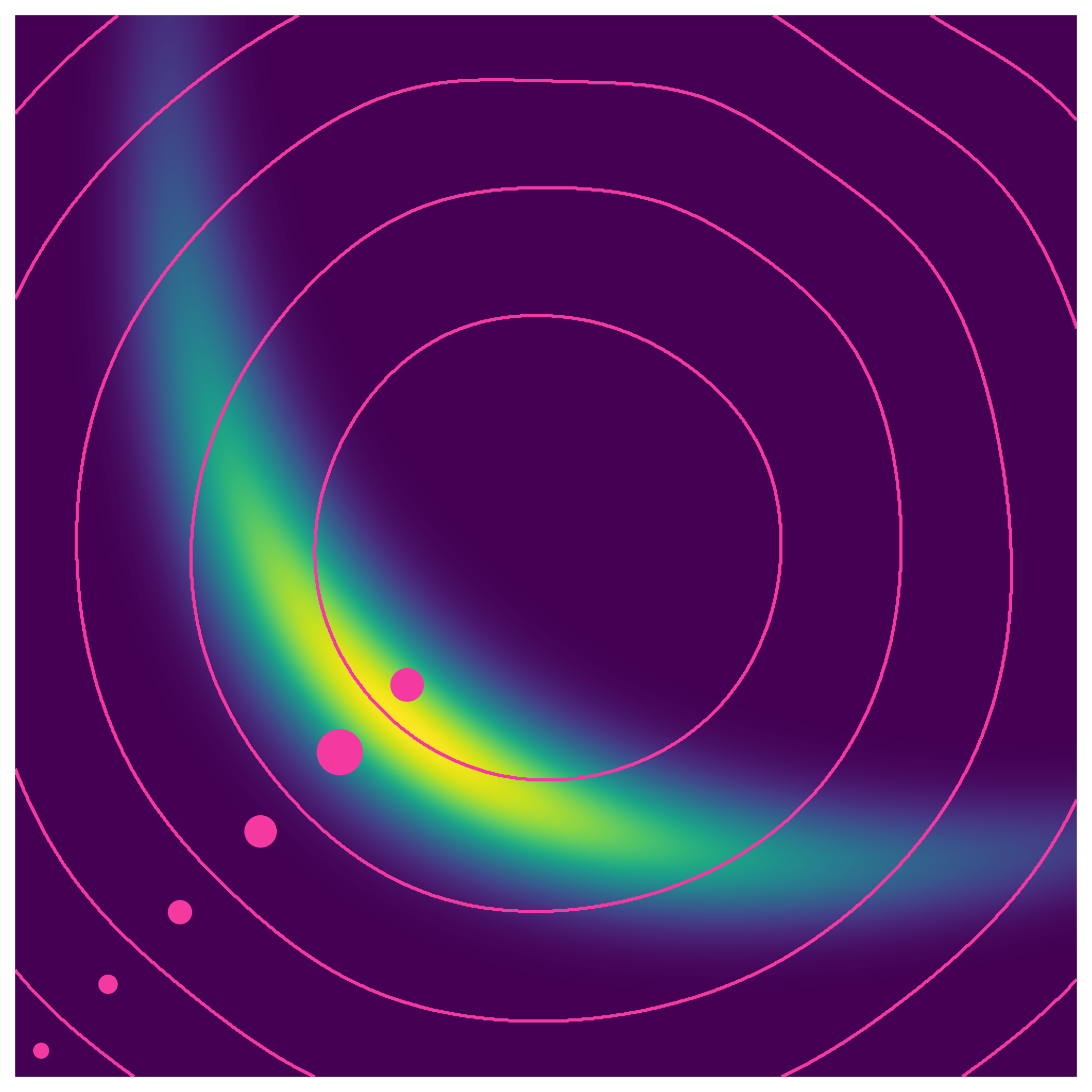
Y. Dubois, B. Bloem-Reddy, K. Ullrich, C. J. Maddison
NeurIPS 2021 Spotlight Presentation 🎉TLDR: We formalize compression with respect to ML algorithms rather than human perception.
Selected Papers Compression, Information Theory, Invariance, Representation Learning, Self-Supervised Learning, Vision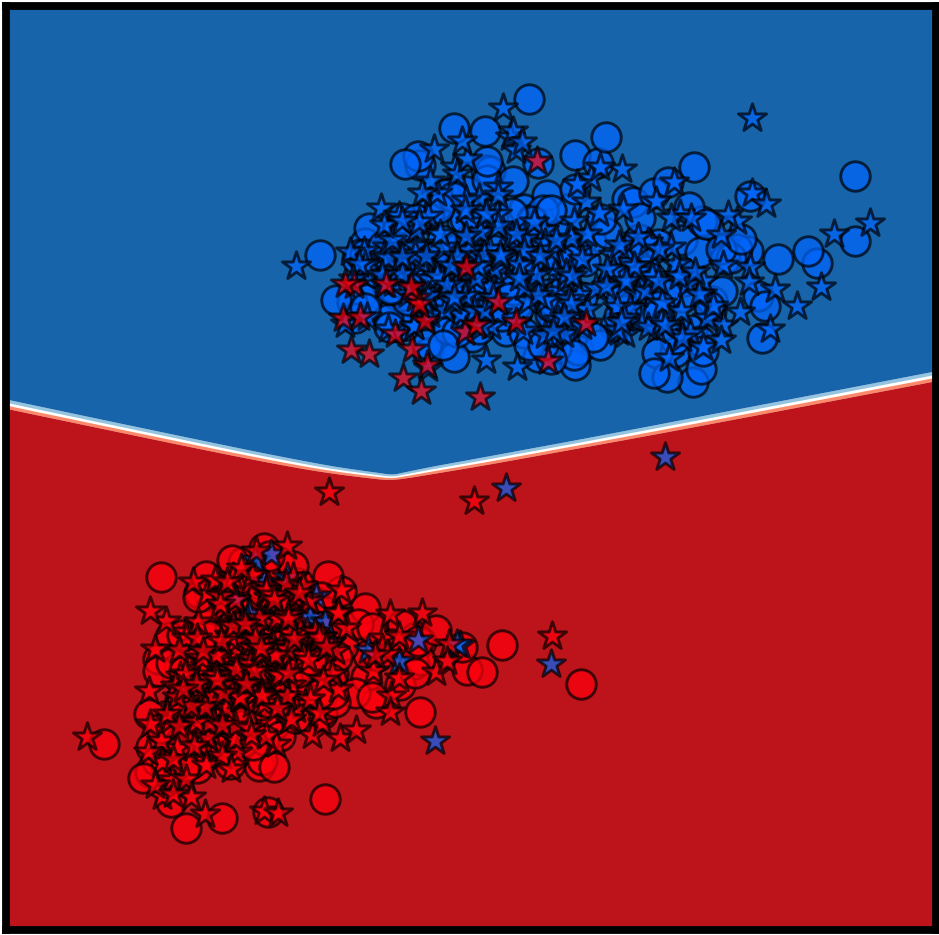
Y. Dubois, D. Kiela, D. J. Schwab, R. Vedantam
NeurIPS 2020 Spotlight Presentation 🎉TLDR: We characterize and approximate optimal representations for supervised learning.
Selected Papers Generalization, Information Theory, Representation Learning, VisionRobustness
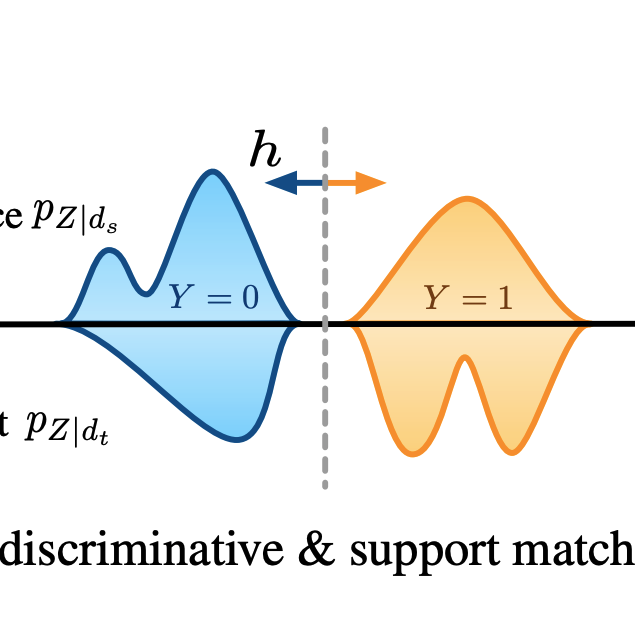
Y Ruan*, Y. Dubois*, C. J. Maddison
ICLR 2021TLDR: We give a simple variational objective whose optima are exactly the set of representations that are robust under covariate shift.
Selected Papers Information Theory, Invariance, Representation Learning, Robustness, Self-Supervised Learning, Vision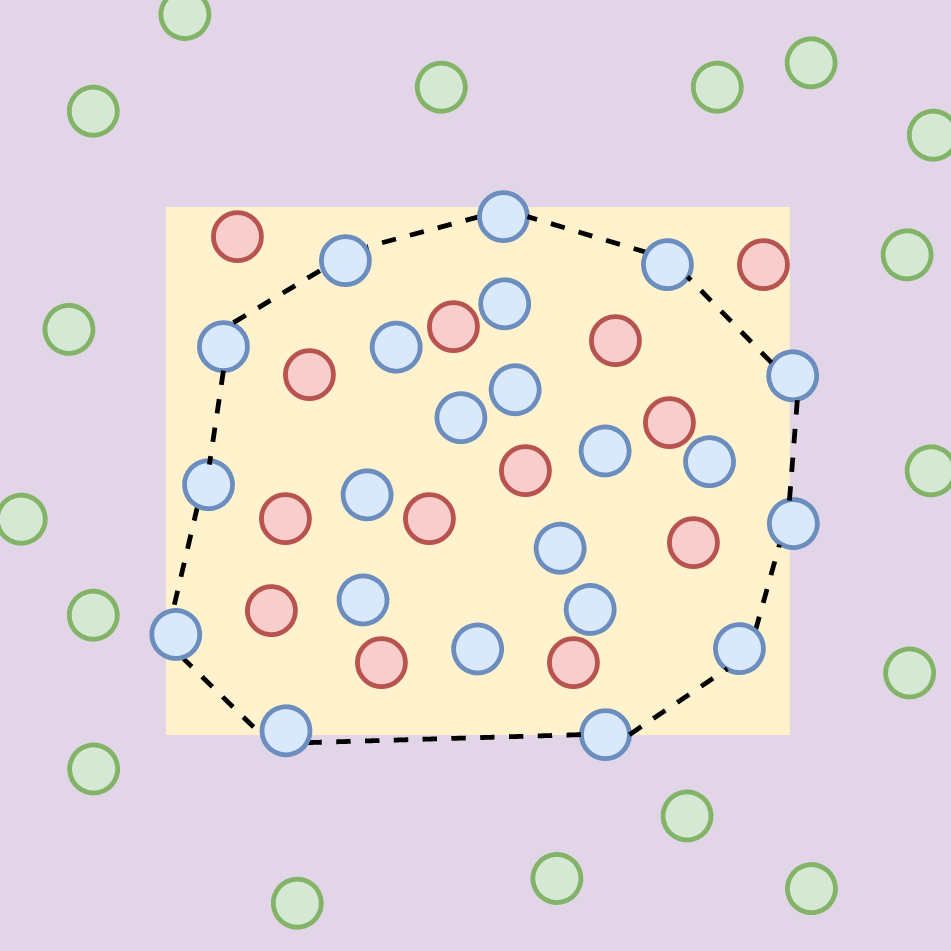
Y. Dubois, Gautier Dagan, Dieuwke Hupkes, Elia Bruni
ACL 2020TLDR: We propose an attention that improves extrapolation capacity of neural NLP models.
Extrapolation, NLP, RobustnessSelf-Supervised Learning
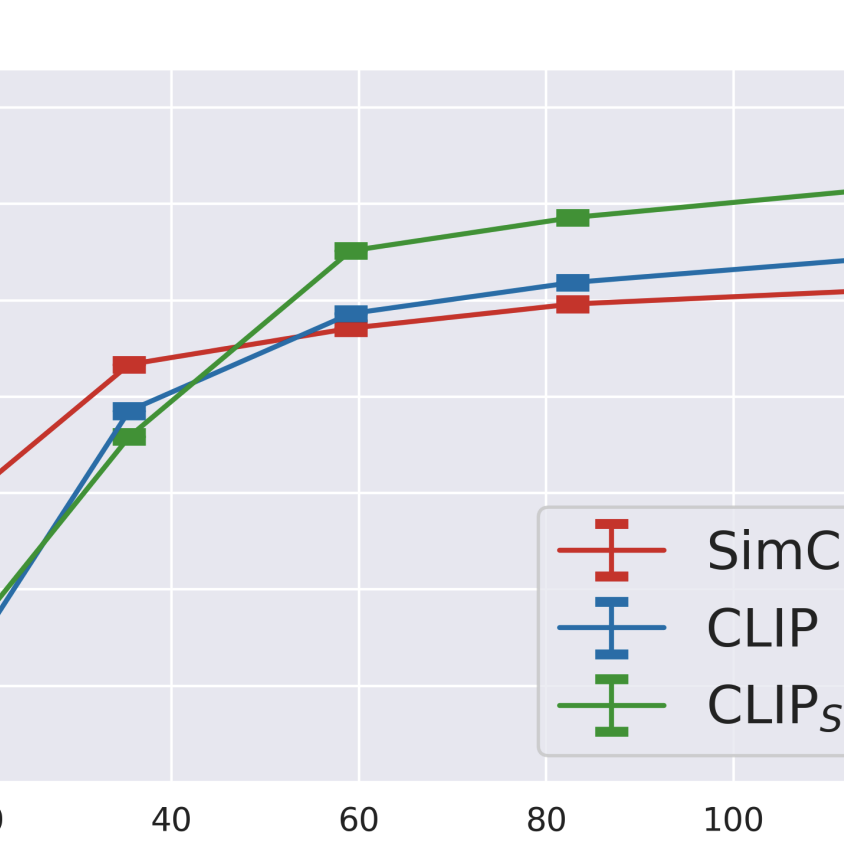
S. Santurkar, Y. Dubois, R. Taori, P. Liang, T. Hashimoto
ArxivTLDR: Our work performs a systematic investigation into whether additional language supervision (in CLIP) helps models learn more transferrable representations.
NLP, Representation Learning, Self-Supervised Learning, Vision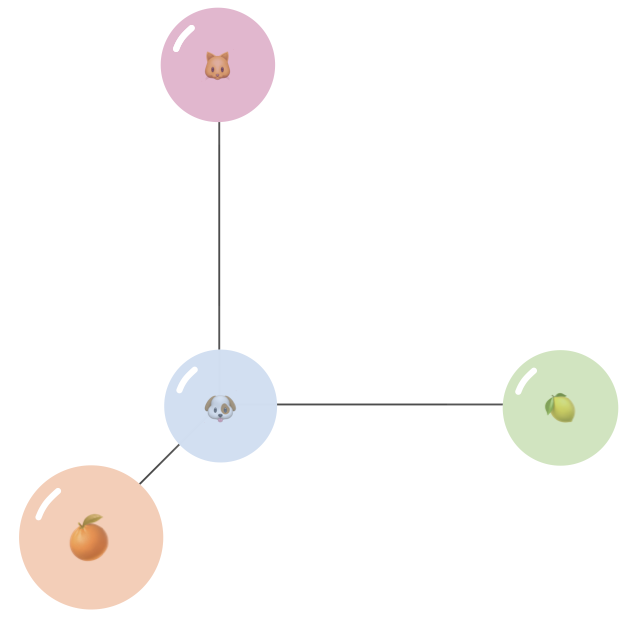
Y. Dubois, T. Hashimoto, S. Ermon, P. Liang
NeurIPS 2022TLDR: We characterize idealized self-supervised representations, which leads to actionable insights for improving SSL algorithms.
Selected Papers Invariance, Representation Learning, Self-Supervised Learning, Vision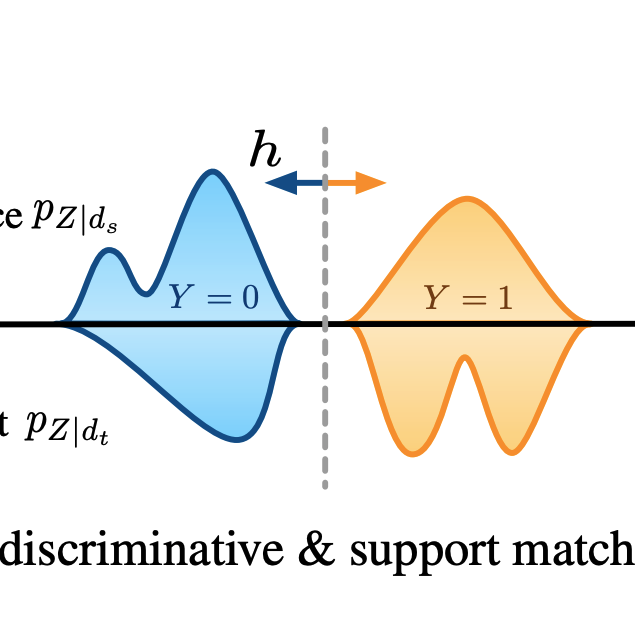
Y Ruan*, Y. Dubois*, C. J. Maddison
ICLR 2021TLDR: We give a simple variational objective whose optima are exactly the set of representations that are robust under covariate shift.
Selected Papers Information Theory, Invariance, Representation Learning, Robustness, Self-Supervised Learning, Vision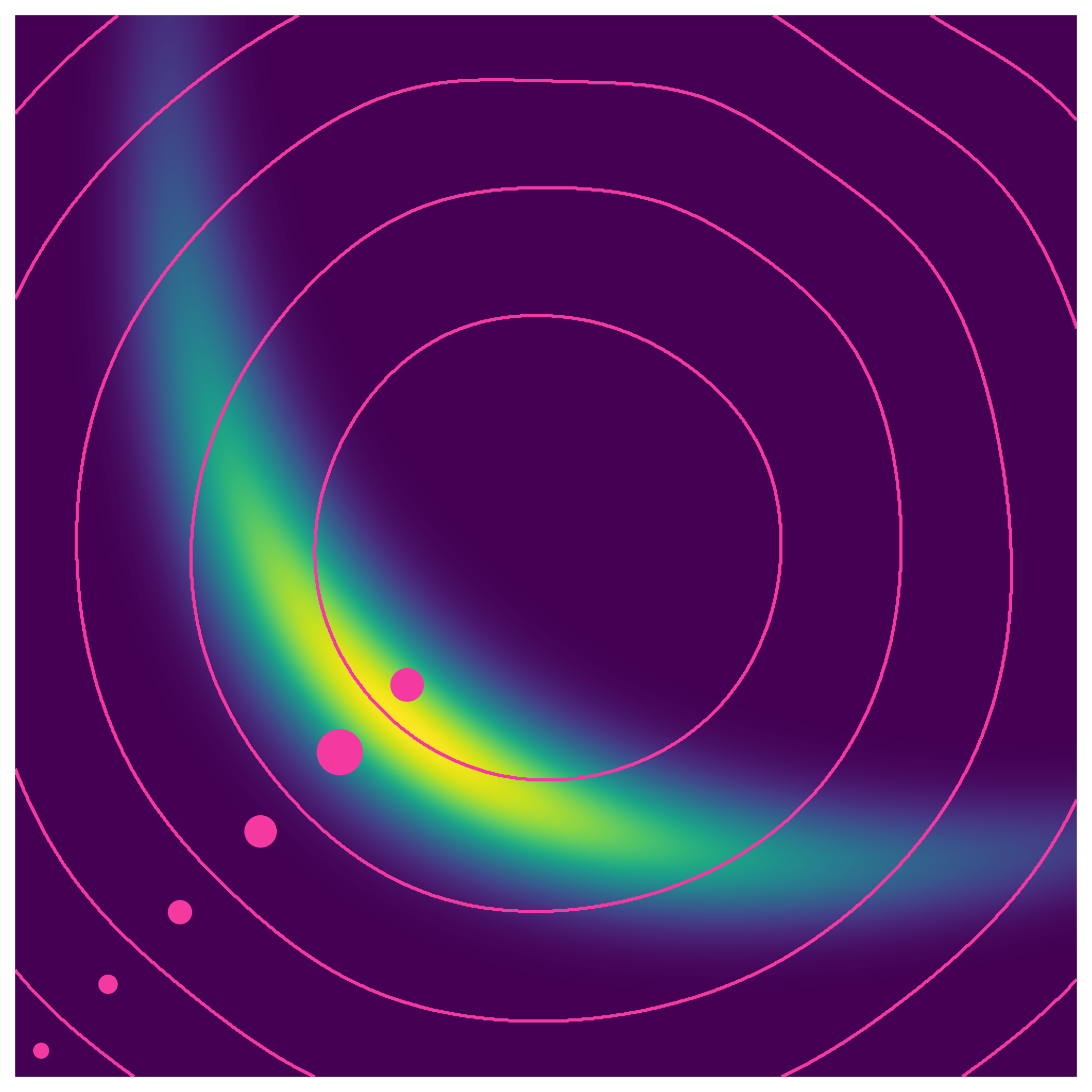
Y. Dubois, B. Bloem-Reddy, K. Ullrich, C. J. Maddison
NeurIPS 2021 Spotlight Presentation 🎉TLDR: We formalize compression with respect to ML algorithms rather than human perception.
Selected Papers Compression, Information Theory, Invariance, Representation Learning, Self-Supervised Learning, VisionTime Series
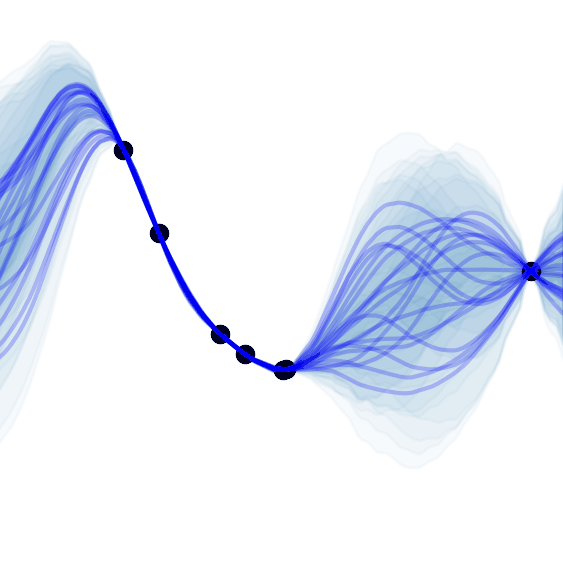
A. Y. K. Foong*, W. P. Bruinsma*, J. Gordon*, Y. Dubois, J. Requeima, R. E. Turner
NeurIPS 2020TLDR: We propose a translation equivariant (latent) neural process.
Equivariance, Extrapolation, Meta Learning, Time Series, Uncertainty, Vision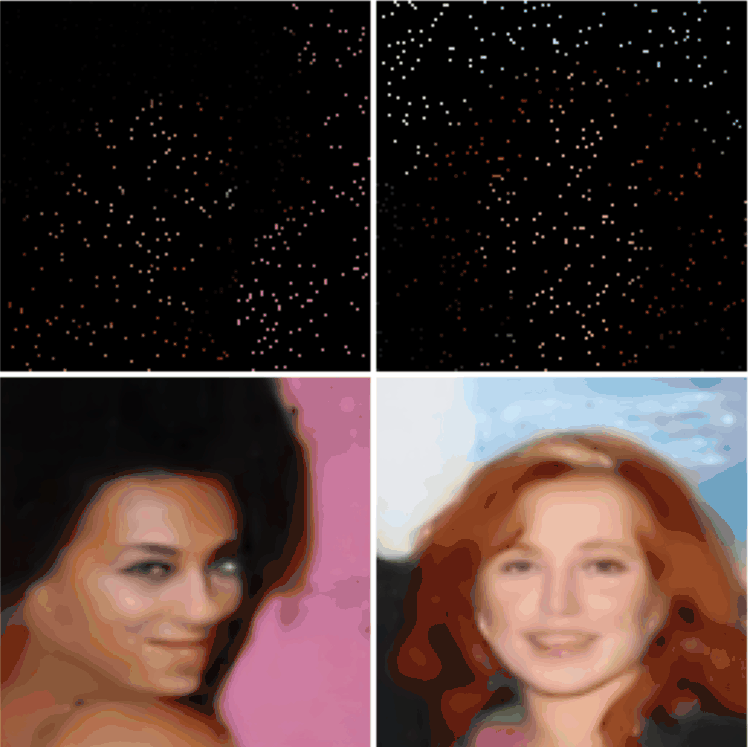
J. Gordon*, W. P. Bruinsma*, A. Y. K. Foong, J. Requeima,Y. Dubois, R. E. Turner
ICLR 2020 Oral Presentation 🎉TLDR: We propose a translation equivariant conditional neural process.
Equivariance, Extrapolation, Meta Learning, Time Series, Uncertainty, VisionUncertainty
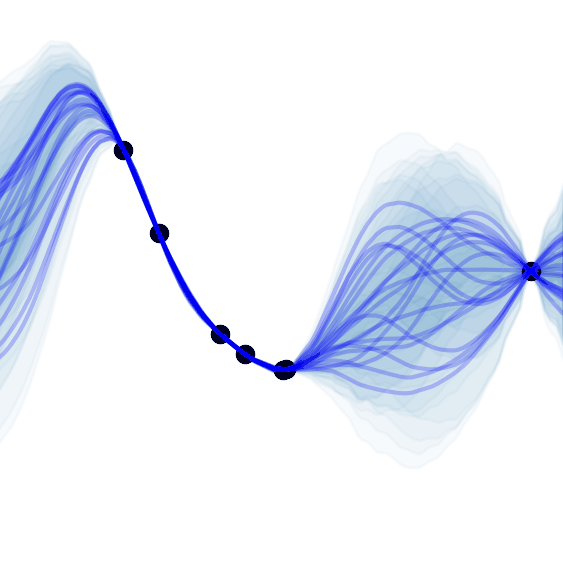
A. Y. K. Foong*, W. P. Bruinsma*, J. Gordon*, Y. Dubois, J. Requeima, R. E. Turner
NeurIPS 2020TLDR: We propose a translation equivariant (latent) neural process.
Equivariance, Extrapolation, Meta Learning, Time Series, Uncertainty, Vision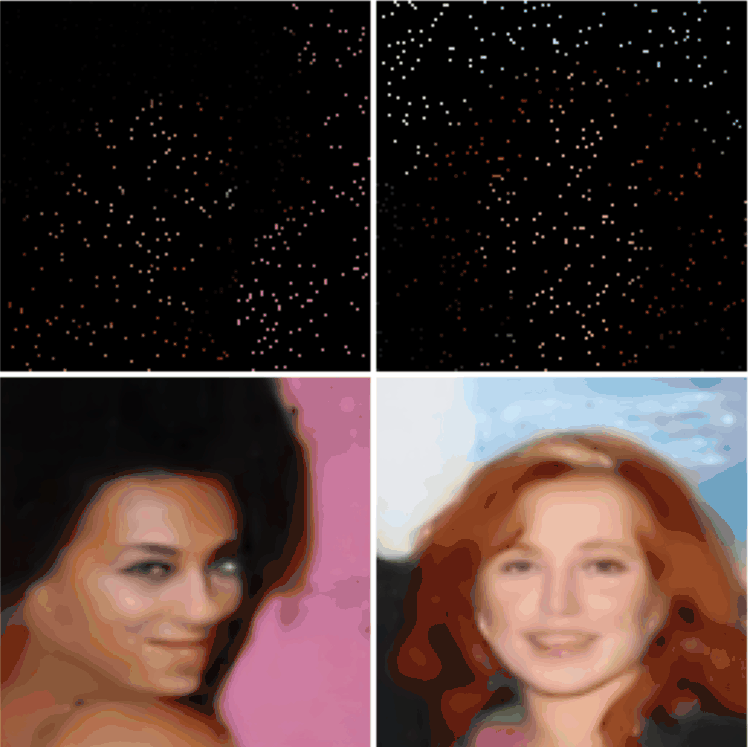
J. Gordon*, W. P. Bruinsma*, A. Y. K. Foong, J. Requeima,Y. Dubois, R. E. Turner
ICLR 2020 Oral Presentation 🎉TLDR: We propose a translation equivariant conditional neural process.
Equivariance, Extrapolation, Meta Learning, Time Series, Uncertainty, VisionVision
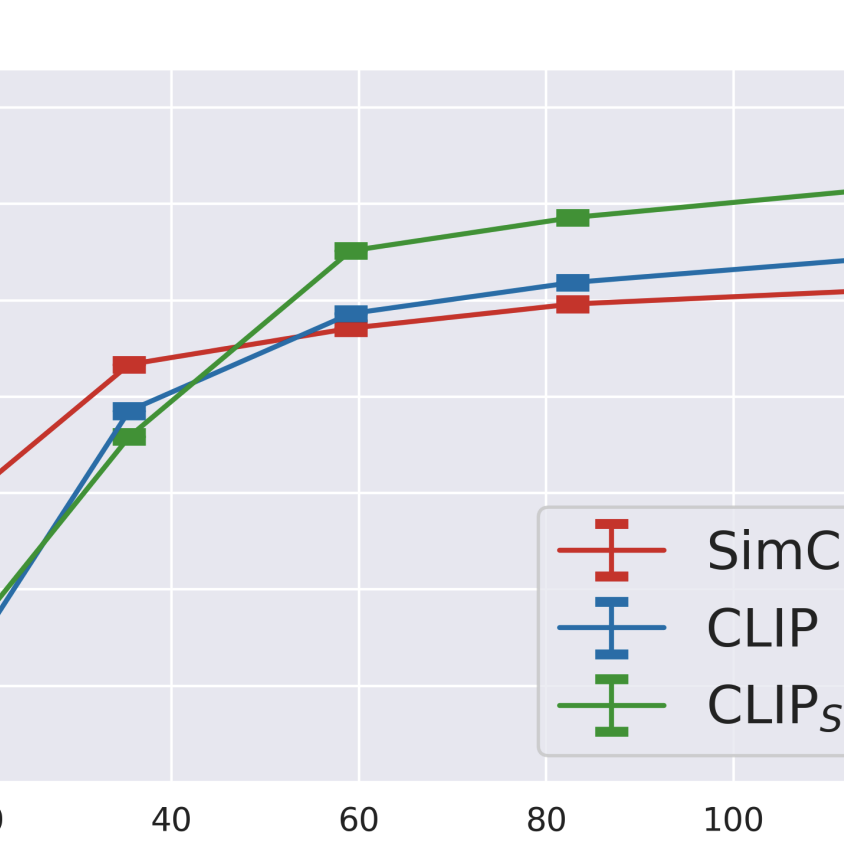
S. Santurkar, Y. Dubois, R. Taori, P. Liang, T. Hashimoto
ArxivTLDR: Our work performs a systematic investigation into whether additional language supervision (in CLIP) helps models learn more transferrable representations.
NLP, Representation Learning, Self-Supervised Learning, Vision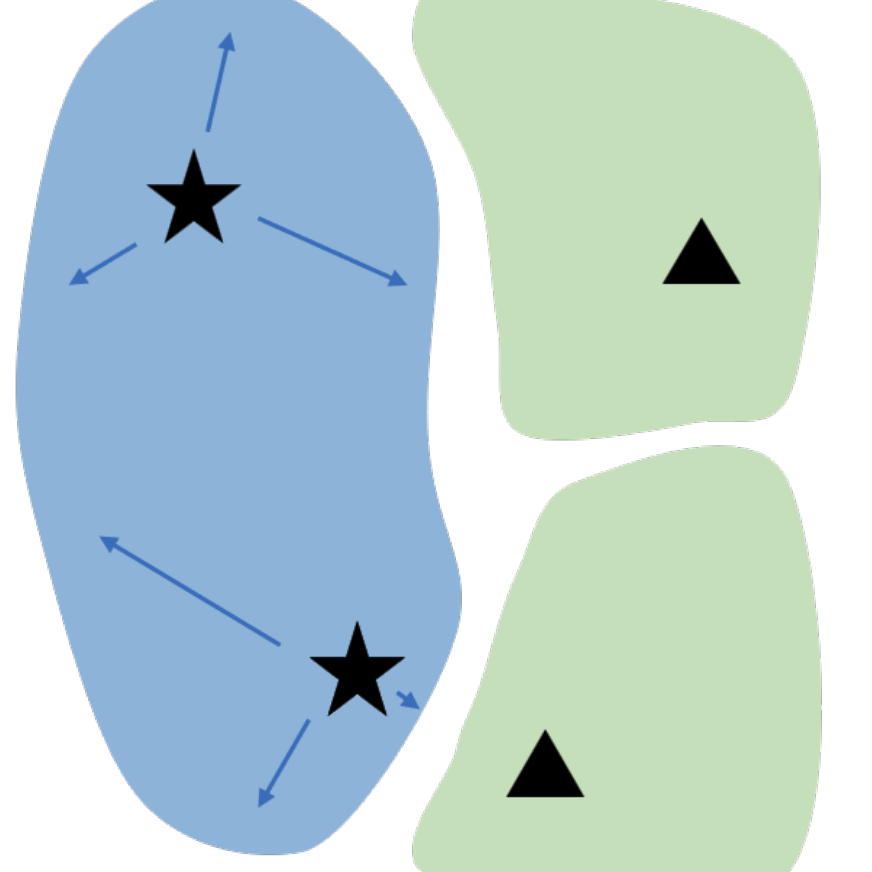
N. Miao, E. Mathieu, Y. Dubois, T. Rainforth, Y. W. Teh, A. Foster, H. Kim
ArxivTLDR: We introduce a method for automatically learning input-specific augmentations from data.
Invariance, Vision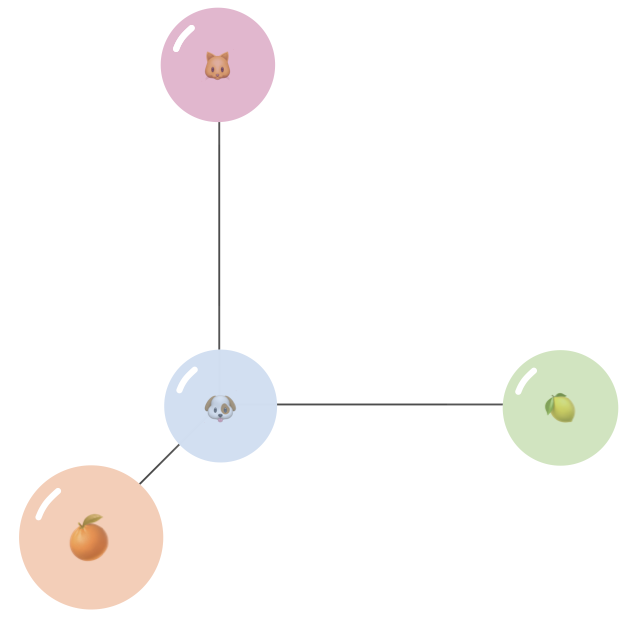
Y. Dubois, T. Hashimoto, S. Ermon, P. Liang
NeurIPS 2022TLDR: We characterize idealized self-supervised representations, which leads to actionable insights for improving SSL algorithms.
Selected Papers Invariance, Representation Learning, Self-Supervised Learning, Vision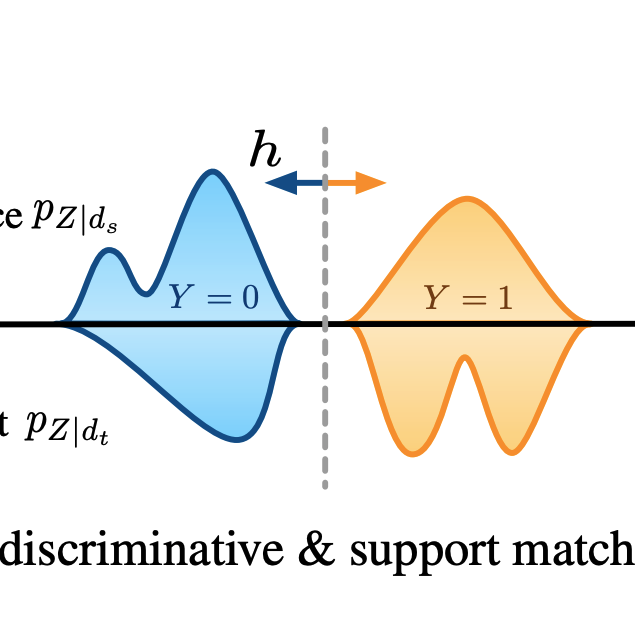
Y Ruan*, Y. Dubois*, C. J. Maddison
ICLR 2021TLDR: We give a simple variational objective whose optima are exactly the set of representations that are robust under covariate shift.
Selected Papers Information Theory, Invariance, Representation Learning, Robustness, Self-Supervised Learning, Vision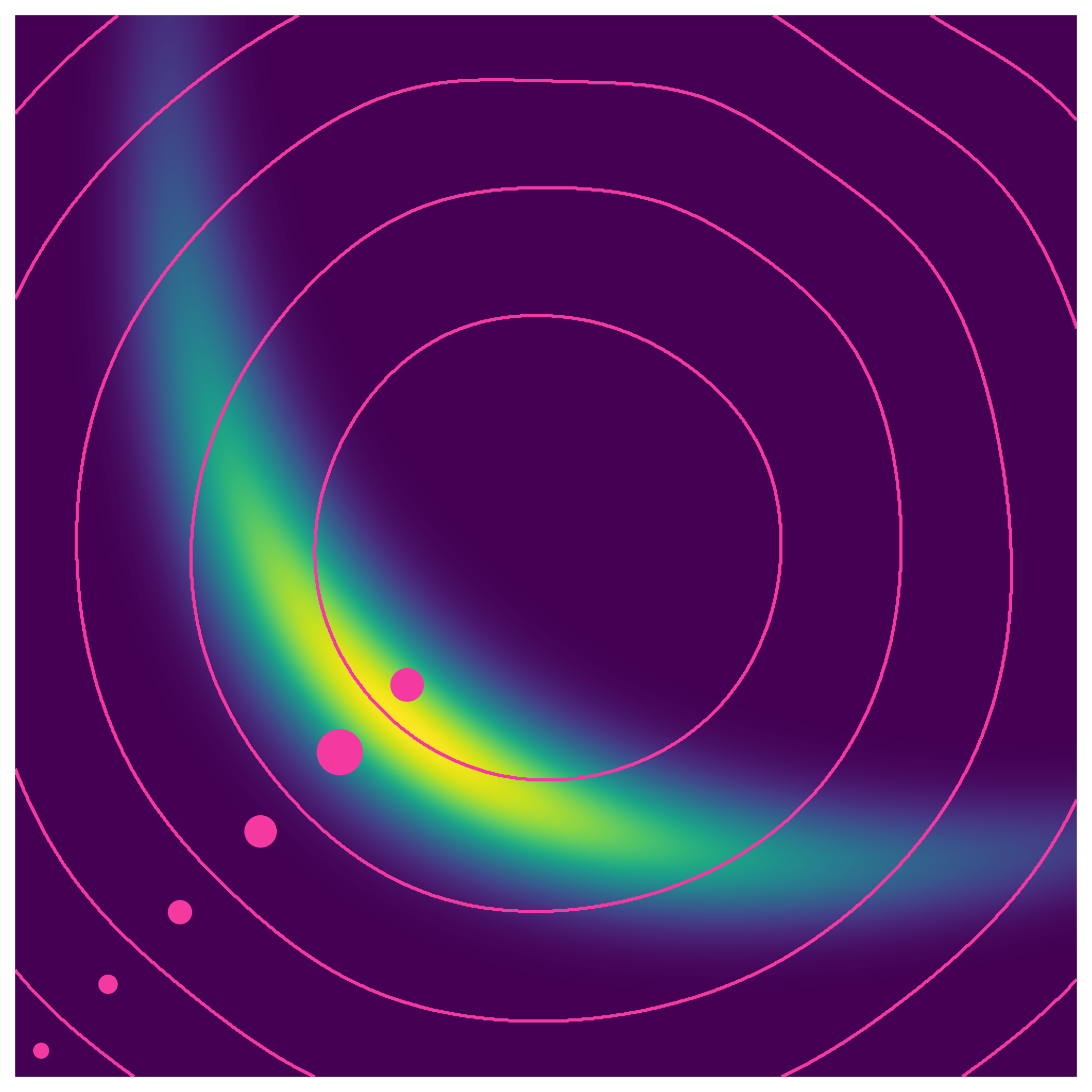
Y. Dubois, B. Bloem-Reddy, K. Ullrich, C. J. Maddison
NeurIPS 2021 Spotlight Presentation 🎉TLDR: We formalize compression with respect to ML algorithms rather than human perception.
Selected Papers Compression, Information Theory, Invariance, Representation Learning, Self-Supervised Learning, Vision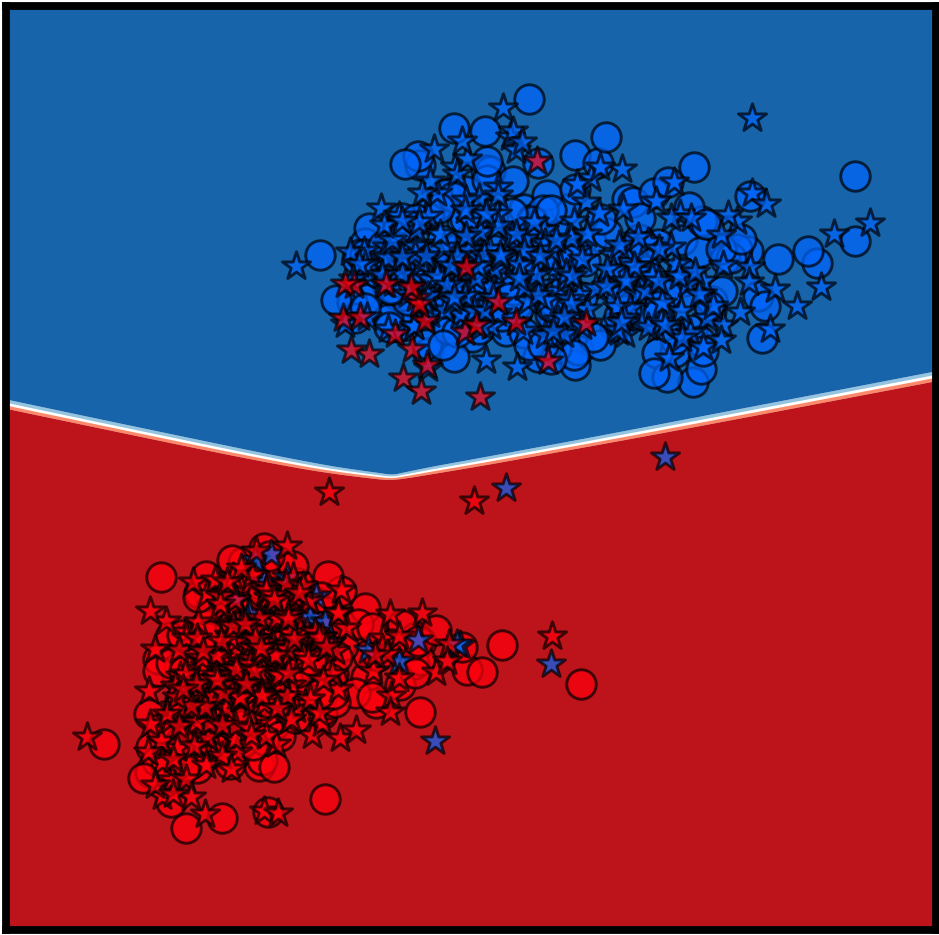
Y. Dubois, D. Kiela, D. J. Schwab, R. Vedantam
NeurIPS 2020 Spotlight Presentation 🎉TLDR: We characterize and approximate optimal representations for supervised learning.
Selected Papers Generalization, Information Theory, Representation Learning, Vision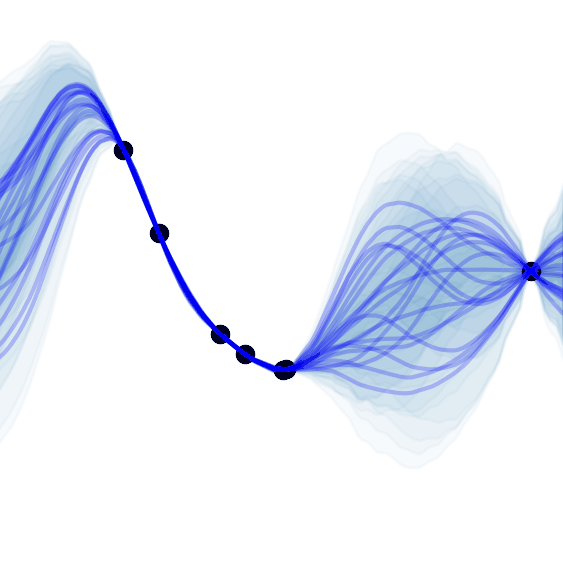
A. Y. K. Foong*, W. P. Bruinsma*, J. Gordon*, Y. Dubois, J. Requeima, R. E. Turner
NeurIPS 2020TLDR: We propose a translation equivariant (latent) neural process.
Equivariance, Extrapolation, Meta Learning, Time Series, Uncertainty, Vision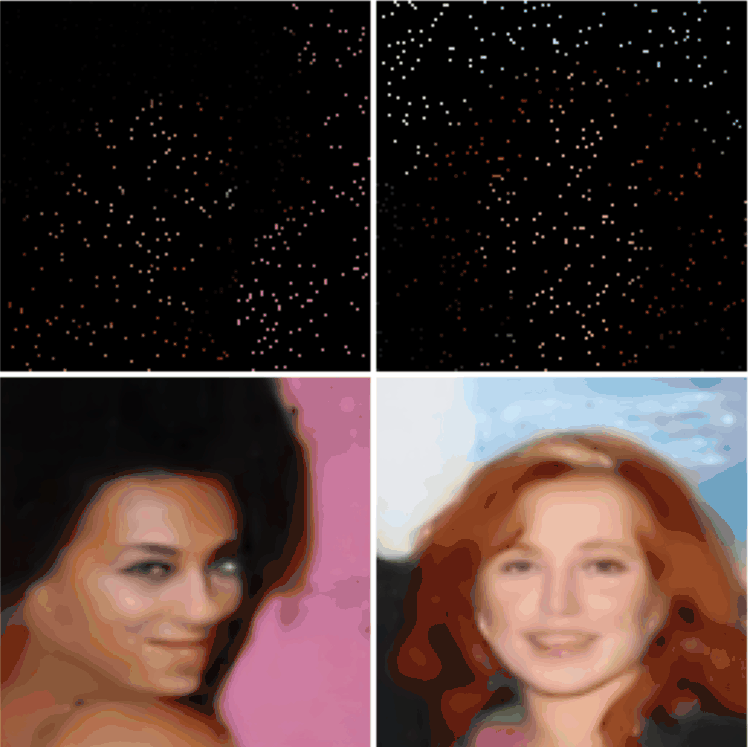
J. Gordon*, W. P. Bruinsma*, A. Y. K. Foong, J. Requeima,Y. Dubois, R. E. Turner
ICLR 2020 Oral Presentation 🎉TLDR: We propose a translation equivariant conditional neural process.
Equivariance, Extrapolation, Meta Learning, Time Series, Uncertainty, Vision